Purpose:
The purpose of this page is show interesting reads from other blogs or other resource that have to do with anything data including data management from the source to the target.
- The article tackles the common question: "Do I need to learn dbt?" The key takeaway is that dbt is a tool, and like any tool, it’s only as useful as the problems it helps you solve. Instead of asking if it's necessary, the better question is: does dbt address your workflow’s challenges? dbt isn't an ELT tool; it's strictly the "T" (Transformation) in ELT, handling data transformations using SQL. It shines in data warehouses where SQL is the standard. The article gives a solid overview of four key problems dbt solves, along with practical examples. Overall, a good breakdown.
No, Data Engineers Don’t NEED dbt.
But It Sure Does Solve a Lot of Problems
- This article gives a decent overview of the crucial role in understanding the significance of data pipelines within the context of data products.
Data Pipelines for Data Products
Key Components, Recommended Tools, and Fundamental Development Concepts
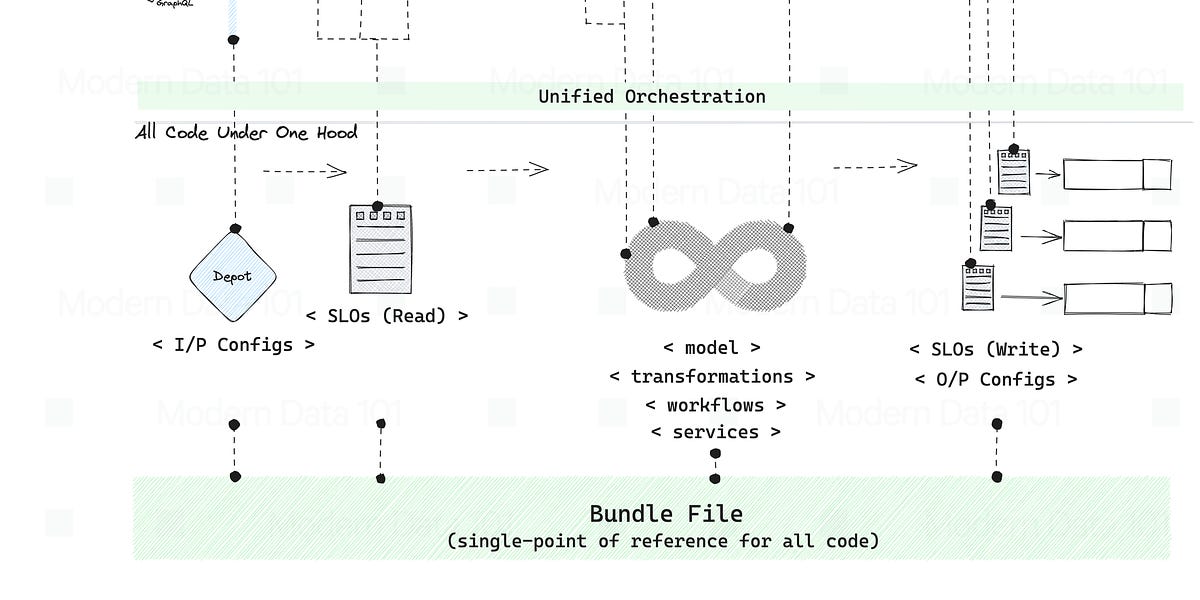
- Data as a product is a mindset that views data as a valuable asset that can be packaged and sold to customers. Data products are specific products or services that are built on top of data, such as a machine learning model or a data visualization tool. In a data mesh, data as a product is the foundation for building data products that meet the needs of different business users.
Data as a product vs data products. What are the differences?
Understand what data as a product is by looking at an example.
- Percona does an interesting comparison of proxies. It's interesting how long HAproxy has been around and is still pretty relevant. I started to use ProxySQL a few years back and find this post interesting.
Comparisons of Proxies for MySQL
After understanding of the environment, the needs, and the evolution that the platform needs to achieve it’s possible to choose a MySQL proxy.
